Machine Learning, Data Science, & Autonomy
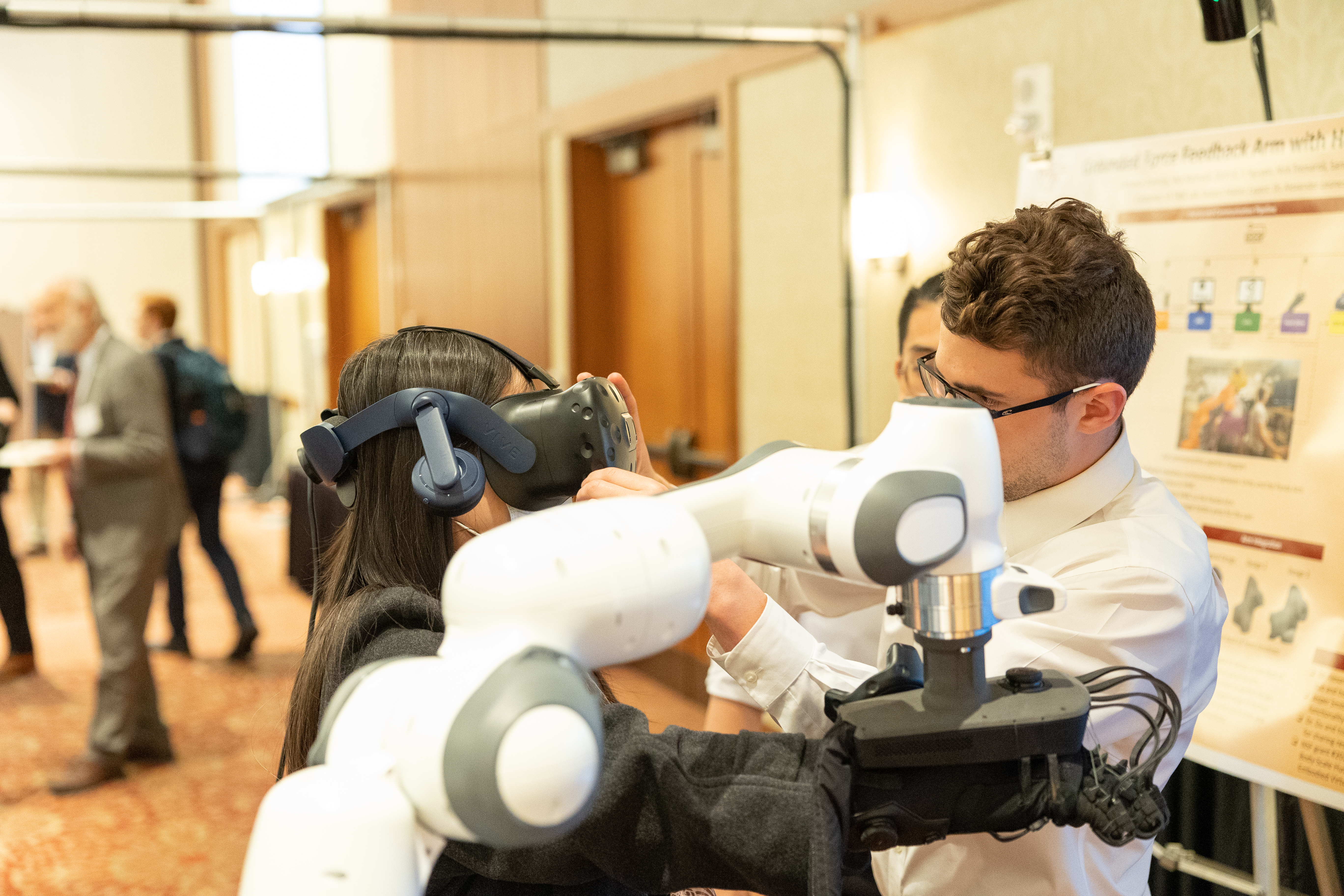
Research in Machine Learning, Data Science, and Autonomy is accelerating the ways computer models and artificial intelligence are used in everything from optimizing cancer treatment to controlling drones. From diving into the complexities of human health to plumbing the depths of the ocean, this research is helping us understand the unknown using these advanced computational tools.
Highlighted Research
For computers to understand what’s happening in a visual scene or image, they need to recognize how humans interact with surrounding objects. We are tackling the challenge of detecting human-object interactions, which will take us a step closer to a fine-grained visual understanding of human activities.
We are analyzing multi-channel electroencephalogram (EEG) data to discern the brain wave patterns associated with ADHD (attention deficit hyperactivity disorder). Using machine learning approaches on a small homogeneous dataset, we have been able to classify ADHD versus non-ADHD with very high accuracy. While this is a promising start, these approaches need to be applied to a much larger, non-homogeneous dataset in order to ascertain usability. Together with colleagues from the Department of Psychology, we are working toward establishing a large ADHD database so that our machine learning approaches can be refined and extended.
Dreaming may be the key to understanding how the brain enables massively higher levels of parallelism than are found in computers, as well as how creative processes occur in the brain. We are focusing on the brain’s ability to build and hypothesis-test potential strategies in nearly perfect parallelism, so that real-time (awake) usage models may be optimized. Computational dreaming (CD) is a meta-algorithm for cognition, and we have developed a maze-solving CD simulator that is about seven times superior to random model selection. Our work shows that CD tends to rediscover known maze-solving algorithms on its own, developing them from primitive moves to complete enumeration combined with a dream phase.
Biological systems usually perform well in the face of significant uncertainty, and ECE researchers are drawing on these systems, especially their control functions, to design artificial systems. We are investigating the theory of active inference, which seeks to explain a wide range of biological phenomena, as a means of controlling nonlinear systems in the presence of uncertainty and stochastic disturbances.
Active inference says that biological systems update a set of internal states that represent beliefs about the environment. The error between observations of the environment and predictions made from the internal states drives this process, updating beliefs about states and predictions, causing the system to act.
Through a grant from the National Institutes of Health, advancements have been made in the understanding and modeling of astrocytes, a type of brain cell. By interpreting the calcium dynamics between these cells, the computational tools can describe and model activity in the brain. This research has already shown that certain brain affecting syndromes actually cause an increase in astrocyte activity compared to unaffected cells. These tools could lead to earlier detection of neurological disorders and pave the way for new treatments or therapies.
ECE researchers are making waves by integrating machine learning and autonomous robotics to shed light on one of the darkest areas of the planet: the ocean floor. Aquatic unmanned vehicles are autonomously scanning and exploring unmapped areas of the seabed with the help of advanced machine learning algorithms which help them navigate the ever changing and chaotic underwater environment.
Cancer is a complex and multifaceted disease, with a constantly changing landscape of prognosis and treatment options. ECE researchers are creating tools and data driven models to provide insight. They are modeling different treatment protocols to improve patient outcomes, and their results suggest that alternating treatments in specific ways can prolong their positive effects.
Combining machine learning with autonomous robotics has led ECE researchers to produce effective, multi-agent drone networks, with each drone feeding information into the algorithm directing the swarm. This technology has applications in search and rescue, topographical surveying, surveillance, and other fields.
Other Research Topics
- Aeronomy of the upper atmosphere
- Prediction of density of the upper atmosphere from measurements of fields and plasma in the solar wind
- Ionospheric electric fields, currents, and heating and effects on the upper atmosphere